What is cryptocurrency mining?
We explain how virtual currencies such as Bitcoin and Ether are actually made
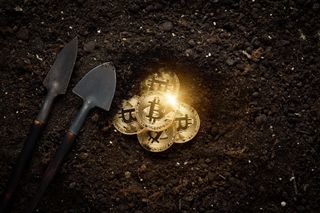
The popularity of cryptocurrencies over the past decade has almost been as turbulent and volatile as their monetary valuations. Having spent many years in relative obscurity, the popularity of cryptocurrencies, or digital tokens, soared in 2019 and 2020, as did the number of cryptocurrency miners. But what exactly is cryptocurrency mining?
The process for creating 'new' cryptocurrencies varies depending on the blockchain in question, however many follow a process known as 'mining'. The process is a cause of concern for many lawmakers and environment groups, as it requires an enormous amount of energy to pull off.
Cryptocurrency mining: A simple definition
Cryptocurrency mining is the name given to the process of completing 'work' on the behalf of the supporting blockchain – the digital ledger technology that supports each individual cryptocurrency. There is one blockchain for each cryptocurrency, controlling all the trading and activity of that particular coin.
When it comes to major cryptocurrencies like Bitcoin, there are only a finite number of new cryptocurrency tokens, or coins, that can be 'minted', which is by design.
Perhaps a good way to visualise this idea is to imagine a group of people stumbling across a large block of gold. There is only a single block of gold, and it has a set weight. The only way for this group to take a chunk of it for themselves is to chip away until they each get a nugget. This is essentially how cryptocurrency mining works – the trading of energy or computational power for a reward.
However, the term 'mining' is a little misleading, as the process does not resemble any sort of mining you may be familiar with.
How is cryptocurrency mining related to the blockchain?
Digital tokens like Bitcoin can’t be printed like money, and the only way to generate new coins is through the process of mining, although the term 'mining' is perhaps misleading. It stems directly from the specific blockchain that powers each individual cryptocurrency, from Bitcoin to Ether.
Get the ITPro. daily newsletter
Receive our latest news, industry updates, featured resources and more. Sign up today to receive our FREE report on AI cyber crime & security - newly updated for 2024.
Each particular distributed public ledger supports the operation of a corresponding cryptocurrency and records all transactions across the extent of its network. For our guide on how blockchain technology works in more detail, check out our explainer.
The blockchain records every time cryptocurrency is traded, with these records being compiled into an endless line of blocks that are all connected. For transactions to be validated, they will need to be verified by other users on the network. This process is key to the way that cryptocurrencies work and avoids instances whereby individuals try to initiate several transactions using the same units.
Cryptocurrency mining is effectively a process of rewarding network users with Bitcoin for validating these transactions.
How are new coins mined?
When a slew of transactions is assembled into a block, it is then appended to the blockchain. However, in order to be rewarded with Bitcoin, a ‘mining’ user, or ‘miner’, needs to perform two tasks: validate 1MB worth of transactions as well as be the first to find out a unique 64-digit hexadecimal number - also called a hash.
Injecting simplicity, speed, and innovation in core insurance processes
The case for Claims Management
Similarly to the blockchain, the network user, or 'node', also holds a record of every transaction. As it is notified, the transactions are validated with a series of checks in order to make sure they are legitimate. The checks include scanning the transactions for a unique cryptographic signature, which is created at the beginning of the process, and confirming whether it is valid or not.
In order to be within a chance of securing new Bitcoin, every miner seeks to validate 1MB worth of these transactions. If successful, they then also have to solve a numeric problem which is otherwise known as 'proof of work'. Users who are able to triumphantly generate the correct 64-digit hexadecimal number, or 'hash', which is either less than or equal to the target hash associated with the block, are then remunerated with Bitcoin.
Due to the difficulty of the task, the only feasible way to find the right hash is to calculate as many combinations as possible, and then wait until a match is found.
In order to stand a chance in being the first one to guess a hash, a user needs to have a high hash rate, or hash-per-second, and the more powerful setup, the more hashes a user can sift through - such are the high computing costs of mining. In order to visualise it, imagine a competition where contestants have to guess the correct weight of a cake. Participants have an unlimited number of guesses and the first one to submit the correct weight wins. In this competition, the winner is most likely to be the contestant who is capable of making the most guesses at the fastest rate.
Proof-of-work vs. proof-of-stake
Different blockchains operate on different models, and the advantages and disadvantages of each have long been discussed by cryptocurrency enthusiasts. Ethereum recently made the decision to move to a different, more modern and greener type of blockchain, but the complexities involved in doing so have resulted in delays to the switch.
Bitcoin’s blockchain uses a proof-of-work model, which involves computers dedicated to performing calculations on behalf of the blockchain and then earning a Bitcoin reward. This model has been heavily criticised for its energy use, mainly because the system incentivises the formation of large numbers of powerful computers that are able to act as one and effectively muscle other miners out of the way and take the rewards for themselves.
Ethereum’s upcoming proof-of-stake model aims to eliminate both the high energy use and dominance of ‘mining farms’. It assigns computational power used for cryptocurrency mining at random and it promises to drastically reduce energy since mining farms will not be able to secure their previously dominant share of mining. In fact, Ethereum predicts moving to proof-of-stake will cut its energy use by 99%.
The limits of cryptocurrency mining
What this means is that mining for cryptocurrencies is a matter of competing in a highly competitive race against other participants, all hoping to land the winning ticket and grab a payout.
Business value on AWS
Four key dimensions that will help to build a comprehensive business case for the cloud
However, that’s not the only challenge you will face if you attempt to mine yourself. The difficulty of calculating each hash also increases artificially in order to maintain a steady flow of newly created blocks. This means that as the block count rises, so too does the processing power required to solve each calculation, and therefore the harder it is to mine as a hobbyist. There’s also the issue of hard limits on total circulation. For example, within the Bitcoin blockchain, there will only ever be 21 million coins created in total, a deliberate design to prevent inflation.
In the early days of each cryptocurrency, it’s perfectly possible for everyday users to participate in mining, but given the issues outlined above, the maturity of cryptocurrencies such as Bitcoin means that it’s no longer possible to use standard PCs. The complication of the calculations involved, coupled with the sheer number of other people on the network, means that Bitcoin mining can now only be done with large scale processing ‘farms’ – multiple specialised GPUs working in tandem on a 24-hour basis.
In fact, these days it’s almost impossible to create a return on investment from mining as the energy costs required to power GPU farms typically outweigh the value of the currency itself. Either that, or you’ll be forced to funnel the majority of what you earn into the running and maintenance of the equipment.
As the Bitcoin hype is more or less fully nestled in the wider public consciousness, organisations have invested increasingly considerable sums into it, effectively industrialising cryptocurrency mining. Large warehouses packed to the brim with floor-to-ceiling racks of expensive graphics cards, working towards the sole aim of mining new units of Bitcoin, Ether, Litecoin, and so on, have become the norm.
The Bitcoin network – to add some context – processes 5.5 quintillion hashes per second, which means that unless you have the equipment capable of processing a massive quantity of calculations in a very short space of time, the chances of you being able to compete with the more industrial operations are minuscule.
For this reason, miners often band together and pool resources to maximise their chances of profiting from the cryptocurrency mining game – creating 'mining pools' – sharing their power, as well as any returns their efforts may generate between them.
The legality of cryptocurrency mining
The legality of cryptocurrency mining differs across countries, and bans are usually imposed for one of two reasons. The first is usually linked to a state’s approach to cryptocurrency overall, meaning that if a government is against cryptocurrency trading, it will likely outlaw mining as well.
An example of this is China, where the State Council Financial Stability and Development Committee banned all cryptocurrency trading and mining in 2021 due to its alleged links to illegal activity. Chinese banks and financial institutions are also prohibited from providing cryptocurrency-related services – a move that sent the price of Bitcoin tumbling by roughly 30% in May 2021. Prior to the crackdown, China was home to roughly 65% of the world's cryptocurrency mining. Today, the country’s share of global Bitcoin mining has fallen to effectively zero, according to the Cambridge Bitcoin Electricity Consumption Index.
The second reason that states ban cryptocurrency mining is linked to its immense energy consumption – Bitcoin consumes roughly 148 Terawatt hours (TWh), which is more than entire countries. For instance, Argentina, the Netherlands, and the United Arab Emirates consume 121 TWh, 109 Twh, and 113 Twh respectively. In January 2022, during the “worst energy crisis in a decade” affecting a number of European countries, Kosovo officials banned cryptocurrency mining in an effort to prevent additional electricity blackouts. Prior to the ban, Kosovo was considered a cryptocurrency mining haven due to its low – and often state-subsidised – electricity bills, with some miners refusing to pay them altogether. However, for the time being, the state hasn’t passed any legislation that opposes cryptocurrency trading itself.
Dale Walker is the Managing Editor of ITPro, and its sibling sites CloudPro and ChannelPro. Dale has a keen interest in IT regulations, data protection, and cyber security. He spent a number of years reporting for ITPro from numerous domestic and international events, including IBM, Red Hat, Google, and has been a regular reporter for Microsoft's various yearly showcases, including Ignite.