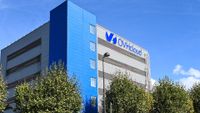
OVHcloud just open sourced its data center liquid cooling system — here’s why
News OVHcloud will open source two components used in data center cooling systems, claiming its tools will help operators with sustainability efforts
Receive our latest news, industry updates, featured resources and more. Sign up today to receive our FREE report on AI cyber crime & security - newly updated for 2024.
By Emma Woollacott published
News Vantage said the development will primarily use biofuels, and needs virtually no water for cooling
By Steve Ranger last updated
News IBM’s acquisition of HashiCorp represents a major signal of intent for the tech giant and its hybrid cloud and AI ambitions
By Emma Woollacott published
News The watchdog wants to examine whether the deals fall within UK merger rules or raise competition concerns
By ITPro published
whitepaper Why and how to gain your customers’ trust with your AI and digital transformation strategy
By ITPro published
whitepaper Five ways to gain new efficiencies and insights
By ITPro published
Whitepaper Securing hybrid cloud infrastructure from alien forces
By Rory Bathgate published
ITPro Podcast Better public and private sector collaboration is needed if the UK’s AI approach is to match the ambition of other nations
By George Fitzmaurice published
Analysis Through its Bedrock and Amazon Q services, AWS has committed itself to AI accessibility at the enterprise level
By Jack Peters published
Industry Insights Data underpins our lives, but many businesses do not appreciate the true value of their data and fail to adequately secure it. Here’s what needs to change
By Steve Ranger published
Case study Using cloud backups, Leo A Daly dramatically reduced file recovery times and improved collaboration across its sites
By Dave Mitchell published
Reviews The DL560 has a unique closed-loop liquid cooling system capable of handling a diverse range of heavy-duty workloads
By Stuart Andrews published
Reviews Good design, strong ergonomics, impressive performance, and a bigger screen make this a brilliant, business-ready Chromebook for long working days
By Stuart Andrews published
Reviews While not the strongest screen quality or performance, the Flex 5i Chromebook Plus gets most of the way there on a lower budget
By Alun Taylor published
Reviews With a potent Nvidia GPU and superb 120Hz OLED display, the new Vivobook Pro is a superb do-it-all laptop that won't break the bank
By Rory Bathgate published
ITPro Podcast Better public and private sector collaboration is needed if the UK’s AI approach is to match the ambition of other nations
By Rory Bathgate published
ITPro Podcast With the EU AI Act’s sweeping requirements on their way, companies will need to take precise steps to demonstrate AI risk management
By Rory Bathgate published
ITPro Podcast Google's data heritage could be just the right ingredient as it takes on the AI market at scale
By Rory Bathgate published
ITPro Podcast A major attack on the electoral commission raised concerns for the security capabilities of public and private organizations
By Dale Walker published
ITPro 20/20 The technology, policies, and markets likely to shape IT decision-making in the year ahead
By Dale Walker published
ITPro 20/20 A look at the shady world of cyber security networking and online communities
By Dale Walker published
ITPro 20/20 Issue 32 looks at the problems that can arise when businesses choose to embrace flashy terminology at the expense of simplification
By Dale Walker published
ITPro 20/20 Advancements in the core technology mean holograms could soon become instrumental in eliminating the worst aspects of hybrid work