The top programming languages you need to learn for 2023
With hundreds of coding languages out there, which ones will earn you the most?
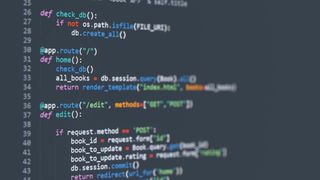
Software and web development have become two of the most attractive career paths in tech thanks to employers increasingly willing to consider applicants without a computer science background, the variety of job opportunities out there, and the generous salary packages.
The IT talent shortage is well documented, and as it stands, there is still a real shortage of software developers fluent in a variety of programming languages. The good news is that jobs are plentiful, secure, and often pay handsomely.
Determining what language to learn can be a difficult task, though, given the number of languages and frameworks available, each serving a different sector of the industry. For example, if you wanted to learn how to program to handle and manipulate large datasets, you wouldn’t choose a language or framework built for mobile app development, like Swift, Kotlin, or Flutter.
The languages in this list have been informed by Stack Overflow’s most recent developer survey, while the salary information comes from PayScale, correct as of June 2022.
Python
£32,400 |
Beginner friendly, incredibly versatile, useful in AI development |
Python is widely considered among the easiest languages to learn, largely due to its simplified syntax, so it's not surprising that it's one of the most popular.
It has become especially popular in the past few years thanks to its capabilities in applications such as machine learning, a fast-growing field. However, it's useful in a range of other areas too such as robotics, AI and big data, so it can be a valuable skill even for experienced devs. What's more, Python was dubbed the 'language of the year' for the second year running by software testing firm Tiobe in 2021.
Python's relative simplicity makes it the perfect skill for new developers to learn, as it provides a great way to get to grips with the basic fundamentals of programming whilst still offering a tangible benefit to earnings potential. Like Java, Python has access to repositories of pre-written codes that will work on most operating systems. This makes it an excellent teaching tool, allowing new coders to think about problem-solving rather than learning a complex language from scratch.
Get the ITPro. daily newsletter
Receive our latest news, industry updates, featured resources and more. Sign up today to receive our FREE report on AI cyber crime & security - newly updated for 2024.
C#
£34,999 |
Great for Windows developers, good option for those working with IoT |
An offshoot of the original C language, C# (pronounced 'C sharp', like the musical note) is a combination of the best features of C and C++. It's built specifically for Microsoft .NET, and is thus one of the best options for developing code for the Windows ecosystem. As such, given Windows' prevalence in the enterprise world, C# developers are rarely likely to be out of work for too long.
The language uses XML and SOAP to include elements of object-oriented programming, which gives developers additional speed when coding. C# is particularly great for developing lean applications; it has a minimal runtime, and it runs incredibly close to the bare-metal it's running on. This makes it a good choice for embedded systems like IoT devices. It's also versatile enough to handle back-end and front-end development.
Java
£38,095 |
Must-have for back-end developers, good for building enterprise apps |
Not to be confused with the similarly-named but unrelated JavaScript, Java is actually a slightly younger and completely different language. While JavaScript is used almost exclusively for web-based programming, Java is more versatile. It's often used for back-end and server-side tasks, for example, and will run across a huge variety of different environments.
It's used by around 9 million developers on some 7 billion devices and PCs, even propping up some of the most viewed websites in the world, including Netflix and Amazon.
One of Java's main benefits is that it's a highly-scalable language, so is well-suited to enterprise applications. It's also been in use for many years, so is already a key part of many organisations' environments.
It was designed to allow programmers to run their code on different machines and operating systems with minimal fuss, so long as there is a Java Virtual Machine (JVM) installed. This allows the code to speak a common language and is an ideal choice for developers working on cross-platform networks.
SQL
£32,583 |
Must-have for database developers |
One of the most venerable programming languages still in mainstream use, Structrured Query Language, known commonly as SQL, was first developed in 1972, and has managed to retain its position as an essential part of modern business IT. This is because it's designed for managing large databases from the likes of Oracle, Microsoft and IBM, which still power a plurality of enterprise applications.
While SQL may seem a bit old-fashioned, it's actually surprisingly versatile, and can run big data applications as well as more traditional databases. It's one of the most reliable languages out there, which explains its enduring popularity.
JavaScript
£26,830 |
The go-to web development language |
Another language that has remained in pretty much constant rotation despite its advancing age, JavaScript powers a phenomenal amount of the web.
Built specifically for web development, it's the technology that powers things like rich media, online video, dynamic pages and much, much more. It also has a number of associated frameworks, such as React, which can be used for other applications like building cross-platform mobile apps.
There are very few pages that don't include various elements of JavaScript, and it's absolutely integral for building contemporary looking and feeling web-based applications. HTML and CSS simply no longer live without JavaScript, or 'JS' as it's so often abbreviated, and it also benefits from being one of the most widely-recommended languages for coding novices to pick up as their first.
Indeed, it's the fundamental foundation of virtually all browser-based SaaS applications, which makes JavaScript programmers highly sought-after.
TypeScript
£50,827 |
Great for large-scale app development, plenty of available jobs |
TypeScript is the Microsoft-created superset of JavaScript, and is among the most popular programming languages in the market right now. It was the fifth most popular in Stack Overflow’s most recent developer survey, which indicates there are plenty of professional opportunities for developers with TypeScript skills, making it an ideal option to expand your skillset.
Microsoft maintains the language, adding new features to it regularly, and it is most often used to develop large, scalable applications. The ten-year-old language was built to be more easily usable than JavaScript, the most popular in this list, and more maintainable too.
Swift
£36,421 |
Must-have for anyone interested in iOS development, solid secondary skill on your CV |
Developed by Apple for creating iOS apps, Swift is an open source language that's designed to be simple to pick up.
Like Kotlin's relationship to Android, Swift is the preferred language for writing apps for any of Apple's platforms, including iOS, macOS, watchOS, tvOS and iPadOS. It has also enjoyed a period of growth in the past few years, in part due to its ease of use and also the increased confidence in its long-term viability which leads to more developers learning the language.
This makes it an essential tool for any app developer, but it does limit its usefulness outside that arena. It's not particularly widely-used within enterprise IT, and it's not an especially versatile language in terms of its applications.
If you're planning to develop for mobile devices, however, it's well worth knowing. It has enjoyed a rise of several places in the past few years in the Tiobe index and may well further increase in popularity in years to come.
C
£38,000 |
Beginner friendly, general purpose, good for OS development |
C is another easy-to-learn, multi-purpose language in this list that is hugely popular in the Windows, Linux, and UNIX communities for its powerful capabilities in system scripting applications. It's a general-purpose language that can be used for a variety of applications in the enterprise all the way through to the gaming space.
C also plays a hugely important role in the heart of most major operating systems, with the kernels of Windows, macOS, iOS, Android, Windows Phone, and most of Linux all written in C. It could be argued much of the world runs on C which makes it an ideal, versatile language to add to your arsenal.
C++
£35,097 |
Highly specialised, good for lightweight app development |
C++ consistently ranks among the most useful programming languages in the world and is an extension of the aforementioned C language. Often relied upon by major system processes like operating systems, graphical user interfaces (GUIs), embedded systems and more recently, VR applications, it's another multi-purpose language that's likely to put you in work for many years to come.
Because the language can make direct changes to the hardware on which it runs, C++ is a great choice for developers looking to build fast, efficient applications that require limited resources. Developers have a significant amount of control over the tuning of their C++ code, making little tweaks to efficiency easy to accomplish.
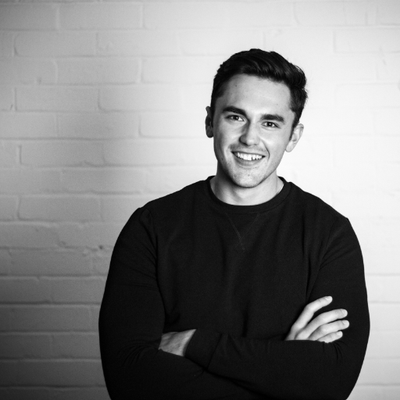
Connor Jones has been at the forefront of global cyber security news coverage for the past few years, breaking developments on major stories such as LockBit’s ransomware attack on Royal Mail International, and many others. He has also made sporadic appearances on the ITPro Podcast discussing topics from home desk setups all the way to hacking systems using prosthetic limbs. He has a master’s degree in Magazine Journalism from the University of Sheffield, and has previously written for the likes of Red Bull Esports and UNILAD tech during his career that started in 2015.