How to pick the best business laptop CPU
With a seemingly endless choice of configurations, understanding the brain of the machine can be key when making a big purchase
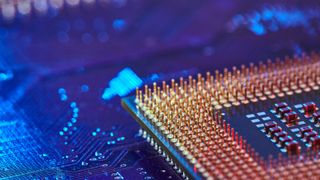
Perhaps the only thing more confusing about buying a new business laptop - or an array of them for an entire workforce - besides the sheer amount of choice on the market, is the myriad different components that equip each model. Laptops vary wildly in terms of technical specifications, so it’s important to understand how each will impact your workers’ ability to get their jobs done.
The central processing unit (CPU), sometimes simply referred to as the ‘processor’, can be seen as the heart, eyes, ears, and brain of the laptop, and is arguably the most important component of the entire device. The confusion here, again, lies in the amount of choice on offer. There are so many different models from different manufacturers on the market, it can feel overwhelming to choose one, especially when the machine will have a direct impact on the quality of work your business is able to produce.
It also doesn’t help that manufacturers don’t make it easy to truly understand what each CPU model will provide you in terms of performance. Many often stick with old hardware conventions, opting for initialisms and numbers in the thousands rather than something a little more explicit. Users often find themselves trawling through comparison websites and social media recommendations before ultimately landing on the CPU they end up purchasing.
That said, there are a few things to know before buying a laptop and understanding what makes a good processor could lead to a better, more productive purchase that stands the test of time, no matter what your business can throw at it.
The best laptop processor
Put simply, there is no straight answer to the question ‘what is the best laptop processor?’ The answer depends on a multitude of factors, many of which relate to the type of work for which you plan to use the business laptop. It will also depend largely on your budget, as laptop CPUs can get eye-wateringly expensive the more ‘premium’ you go.
Before buying a laptop, you need to have a strong idea of what you need the machine to do. Running software for general office work like Microsoft Office 365 is the base standard level of functionality most businesses expect, but some will demand more depending on the job role. For example, you’re unlikely to want to equip an office administrator with the same device as a senior software engineer because the computational demands of a programmer will most likely far exceed those of a more general office worker.
As an easy rule, the best laptop CPU for your business is almost always going to be the most powerful chip you can afford. So, set a budget, understand what you need from the device, and take things from there. But before you go shopping, knowing the differences between manufacturers is hugely important as it could affect performance and compatibility in the long term.
Get the ITPro. daily newsletter
Receive our latest news, industry updates, featured resources and more. Sign up today to receive our FREE report on AI cyber crime & security - newly updated for 2024.
Is Intel or AMD better?
There are two main manufacturers of laptop CPUs on the market today and they are AMD and Intel. Like the allegiances held among devout Xbox and PlayStation console owners, the fanbase for each company can be equally loyal at times, and which is better is ultimately a matter of preference and timing.
Timing is key when making a business laptop purchasing decision, especially when it comes to CPUs. This is because the two companies have been locked in battle for years, each being considered the best manufacturer at different times. Historically, and now again in the present, Intel is considered to make the faster CPUs thanks to its latest 12th-generation Alder Lake chip architecture. However, AMD stole Intel’s crown for a few years recently with its Ryzen 5000-series line of CPUs. These were considered to be vastly superior chips to Intel’s 10th and 11th-generation chips at the time, so if you’re planning to buy an older laptop, or a used machine, then it would be best to check the dates and model numbers to ensure you’re not getting a sub-optimal setup.
CPU naming conventions can be confusing but, fortunately, when it comes to filtering out the Intel chips to avoid, the company’s model numbers clearly specify which generation of chips the model comes from. Each model number starts with the number corresponding to the generation of chips it's from, so an ‘Intel Core i7-12650HX’ processor tells you it’s from the latest 12th-generation line of chips because the model number begins with 12.
A quick way to differentiate the two manufacturers in today’s market is to say that Intel offers better performance, but AMD’s CPUs are more power-efficient and won’t run as hot - generally speaking. Regardless of which manufacturer you choose, you’ll get a highly capable device thanks to modern technology, but if you want the very best on the market, Intel takes the top spot… for now, anyway.
How to choose between an Intel or AMD processor
Intel
Intel’s consumer-grade CPUs come in four main tiers:
- Not the least powerful chip Intel produces but the least powerful we recommend for business use. This is functional for basic office software but not anything more computationally demanding, like photo editing software, for example.
- The happy middle ground that will suit most businesses. Laptops equipped with an Intel Core i5 will breeze through basic software and run more demanding programs well in some cases, but others may experience some lag or stuttering.
- The i7 range is a favourite among the enthusiast crowd and is powerful enough to handle almost any software a business could need with ease. These models are typically much more expensive than an i3 or i5, so they should only be considered if your budget is large or if there is a genuine business need for such performance.
- A more recent addition to the consumer-grade lineup, the i9 is unlikely to be found on many business-focused laptops, but is one of the best models available if your business needs laptops to run many computationally demanding tasks all at the same time, for example.
AMD
Handily, AMD’s naming structure for its flagship line of chips is very similar to Intel’s, in that the most affordable series is indicated by the number 3 in the Ryzen 3 line, and the best performance is offered by the Ryzen 9 chips. It’s worth noting that the most recent Ryzen 6000 chips eliminate the 3-series, however, offering just Ryzen 5, 7, and 9.
Each tier can broadly be characterised in the same way that Intel’s can, too, which is to say the Ryzen 5 is like the i5 - they are both the happy middle ground, while the Ryzen 7 and i7 are both high-end chips that will run almost anything well. The full list of AMD’s consumer-grade CPUs that we’d also recommend for business are as follows:
- AMD Ryzen 3
- AMD Ryzen 5
- AMD Ryzen 7
- AMD Ryzen 9
If you’ve already done some research into AMD Ryzen chips, you may have encountered the company’s AMD Ryzen Mobile Processors for Business line of chips. These may appear to be different to the normal Ryzen Mobile CPUs, but the chips themselves are the same.
Intel vPro vs AMD Ryzen Pro
However, there are also AMD’s Ryzen Pro Mobile Processors for Business, and Intel has its own equivalent line called vPro. These chips are almost identical in terms of performance, but are aimed at businesses who want additional features that could improve things like cyber security and management, for example.
Both Intel vPro and Ryzen Pro use the same model naming schemes as described previously, but offer features like additional security layers to prevent cyber security vulnerabilities. Both AMD and Intel have worked with Microsoft to offer Secured-Core PCs which protect against firmware-level cyber attacks and offer extra provisions for securing sensitive data - somewhat important given today’s data regulatory climate.
The extra features that come with these lines of chips could be hugely beneficial to your business so it’s worth having a conversation with your IT team to decide if they are worth the extra money, and the time it would take to configure for an entire workforce.
Business laptop CPUs to avoid
Intel and AMD also have other consumer-grade CPUs on the market but no others we would feel comfortable recommending for business use. Intel has its Pentium and Celeron lines which, in our opinion, do not offer enough performance to run a business machine seamlessly, and all the software it typically requires. Equally, AMD’s Athlon CPUs will offer performance that will likely leave your workers needing more to get the job done well.
The potential savings to be made by choosing a lower-spec model over the ones we recommend can seem attractive, but when you consider how long you want the machine for and factor in degrading performance over time, it’s better to invest more at the outset rather than having to replace the laptop a year down the road.
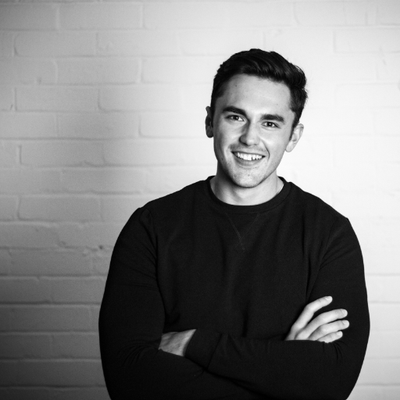
Connor Jones has been at the forefront of global cyber security news coverage for the past few years, breaking developments on major stories such as LockBit’s ransomware attack on Royal Mail International, and many others. He has also made sporadic appearances on the ITPro Podcast discussing topics from home desk setups all the way to hacking systems using prosthetic limbs. He has a master’s degree in Magazine Journalism from the University of Sheffield, and has previously written for the likes of Red Bull Esports and UNILAD tech during his career that started in 2015.