Cloud storage: How secure are Dropbox, OneDrive, Google Drive, and iCloud?
Each of these popular cloud platforms boasts robust security - but which does it best?
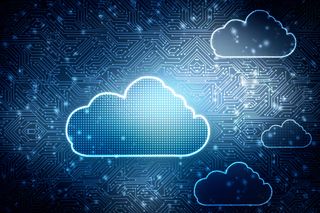
Cloud computing has grown in recent years to become one of the dominant forces within the world of business IT. Its importance has only been cemented over the past 12 months due to the COVID-19 pandemic, which led to a boom in employees working outside of the confines of the traditional office. As such, we have become more dependent on cloud-based services than ever before, relying on them to store both work and private data.
Address multi-cloud configuration risks
Cloud security challenges and how to overcome them
Understandably, the security of these platforms has become a key concern. Hacking and ransomware attacks have spiked during the pandemic, and traditional cyber security solutions based on suddenly outdated office-based working models are not sufficient to protect our dispersed workforces. A report in October revealed that ransomware attacks in the UK were up by 80% during the pandemic as hackers took advantage of the new vulnerabilities created by mass remote working.
The quality and security offerings of the various cloud platforms and services will undoubtedly be at the forefront of IT decision makers’ minds. So which are the best? We’ve broken down the leading providers, assessing their security protocols to help you work out which will keep you best protected. You can check out our companion piece too, which offers an general overview of the pros and cons of each service.
How secure is Google Drive?
Google Drive has become a go-to storage platform for businesses, partly due to how well it integrates with third-party apps and its seamless connection to other Google services. However, this has created a concern around how much access it has to other services and platforms should it be hacked into.
Thankfully, Google has used HTTPS on all its services for years and also has a team dedicated to monitoring compromised account activity. On top of that, Google also uses two-factor authentication and SSL encryption for data transferring to and from a device – it does, however, use the weaker 128-bit AES encryption for data at rest.
History of Google Drive hacking
Given that Google’s cloud services are so interwoven, a hack on one service tends to put the others at risk. In 2014 it was claimed that nearly five million Gmail accounts had been hacked when a database of user credentials was found on a security forum on a Russian website - although this turned out to be a dump of older phished passwords that had largely been reset by Google in the time since the theft.
While Google Drive itself has never fallen victim to a major cyber security incident, a system administrator recently flagged a flaw in the cloud storage system which they claimed could be used by a hacker to trick users into downloading malware or ransomware. The flaw related to Google Drive's "manage versions" feature, which lets you upload a new version of an already-uploaded file.
Get the ITPro. daily newsletter
Receive our latest news, industry updates, featured resources and more. Sign up today to receive our FREE report on AI cyber crime & security - newly updated for 2024.
How secure is Dropbox?
The second platform on our list enjoyed popularity among consumers as an easy-to-use file storage suite, although it has shifted towards the enterprise market in recent years.
During that time, Dropbox has also improved its security protocols in response to growing threats online, including the encryption of data in transit using secure sockets layer (SSL), and at rest using AES-256 bit encryption. The platform also has stolen and lost device protection, allowing you to unlink devices from your account on the fly.
Business users get some extra features, including the option to set permissions for file collaboration and enable password protection and expirations to any shared links.
Dropbox employees are unable to view the content of your files, although the company does have the mechanism to access files if required to do so, such as during a legal investigation. Metadata is accessible by employees too, normally as part of tech support.
History of Dropbox hacking
Major Dropbox hacks have been few and far between, although those that occur proved to be particularly damaging for the company.
The first happened in 2012 when a compromised password was used to access a Dropbox account owned by an employee. At the time, Dropbox said the hack provided an intruder with access to documents containing a handful of customer email addresses, which became the target of phishing attacks. This prompted Dropbox to add two-factor authentication to account logins.
However, in 2016 it was revealed that the hack was much larger than previously thought, with a dumped database of 68 million passwords being leaked online that was said to stem from the initial 2012 breach. Dropbox said at the time that there were no indications that user accounts had been compromised following the incident.
How secure is iCloud?
Apple has built up a reputation for excellent security. Although its iCloud platform had its reputation tarnished briefly when it fell victim to a high-profile hack in 2017, the service continues that trend by offering users a robust set of security features.
"iCloud is built with industry-standard security technologies, employs strict policies to protect your information and is leading the industry by adopting privacy-preserving technologies like end-to-end encryption for your data," Apple's iCloud web page promises.
Like Dropbox, iCloud uses SSL to encrypt data in transit, although it uses AES 128-bit encryption rather than the more secure 256-bit used by Dropbox. The only exception to this is in the iCloud keychain, used to store and transmit passwords and other sensitive user data, which uses 256-bit encryption.
However, privacy has become a focus for the company in recent years, making a big deal out of the fact that encryption keys are created at the device level and that Apple can’t access these itself, or any of the data that you might need to decrypt them.
Like many other platforms, iCloud provides security tokens for added authentication when accessing other apps through it, as well as two-factor authentication at login.
History of iCloud hacking
iCloud has actually maintained a solid track record when it comes to security, although one incident served to tarnish its reputation.
In 2017, iCloud came under intense scrutiny after hackers breached around 50 accounts belonging to celebrities and leaked their contents online. Although the incident was actually the result of successful phishing attacks against a select group of celebrities, the integrity of Apple’s cloud platform was called into question. Even now, the 2017 iCloud hack remains one of the most famous data leaks in history.
How secure is OneDrive?
The last entry on this list is Microsoft’s OneDrive, which has largely managed to remain out of the headlines when it comes to security incidents - although Microsoft’s other services, particularly Windows, are some of the most attacked platforms on the market.
That doesn’t necessarily mean it’s more secure than the other platforms. It generally uses the same standards as others, including data encryption, only with OneDrive this is done by syncing your data to the BitLocker on your hard drive. This means that data is encrypted at rest using the BitLocker, while Microsoft Cloud handles encryption while in transit. An additional bonus of this system is that encryption is done on a per-file basis, meaning that if a key was compromised hackers would only be able to access that particular file.
As you might expect, users also get two-factor authentication at login.
History of OneDrive hacking
Unlike the other platforms, OneDrive has never really been targeted by a major data breach, and most security concerns surrounding the platform usually stem from user error, such as accidentally sharing files with someone they shouldn’t have or using weak credentials.
Microsoft has taken steps to remove as many of these issues as possible, and is one of a number of companies championing passwordless logins.
Cloud storage security: A summary
It's a widely accepted fact that no cloud storage system will ever be 100% secure, especially given that upholding the integrity of every account is reliant on the user following best practices.
The decision you have to make as a customer is deciding which storage platform does the most to avoid potential security incidents. The factors that influence this decision will vary depending on the nature of your business and whether you have specialist requirements, such as businesses in a heavily regulated industry.
However, for most consumers and small businesses, each of the platforms listed here are generally good enough for protecting data, as each provides some form of data encryption at rest and in transit - which is perhaps the most important thing here. Data protection is also improving all the time, and each of these platforms are being updated with better safeguards each year, meaning you can typically rely on the company to do most of the legwork.
However, if you’re unsure, you can always encrypt data yourself before you share it with an online platform. That way, even in the unlikely event that a company’s encryption keys are decrypted en-masse, only you will be able to access your files.
Perhaps the most cost-effective way to ensure your data never gets leaked is to follow best practice security principles. Scrap all those reused passwords, invest in a password manager, and take advantage of two-factor authentication if you’re given the option.
Dale Walker is the Managing Editor of ITPro, and its sibling sites CloudPro and ChannelPro. Dale has a keen interest in IT regulations, data protection, and cyber security. He spent a number of years reporting for ITPro from numerous domestic and international events, including IBM, Red Hat, Google, and has been a regular reporter for Microsoft's various yearly showcases, including Ignite.