What is big data analytics?
We explain what big data analytics is, and how the methods of looking at data differ at scale
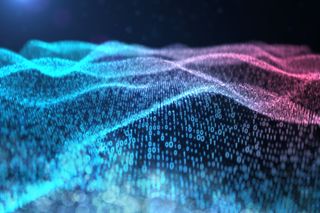
"Big data" seems like a simple term for the way we power modern life, but it is far more complex than that suggests. Essentially, it's a mass collection of information that we use to make decisions, train models, enhance public-facing technology and much, much more.
The methods used to collect the raw data used in big data analytics range widely; the rise of the internet of things (IoT), cloud computing, and increased smartphone use all enable the harvesting of information. For some, such as bad actors, this is used for ill-intent such as identity theft. For businesses, however, data harvesting forms a cornerstone in their quest for increased profit.
Analytics are used to identify the insights, patterns and strategies present within datasets. Specialist software or systems tailored to the task are often used to process high volumes of data quicker than any team of people could. These are then used to inform business decision-making.
What is big data?
To appreciate big data analytics, you first need to comprehend what's being examined.
Big data is defined by three 'Vs' - volume, velocity, and variety. Huge tracts of information are produced every second of the day, and depending on one’s focus may be represented in any number of formats.
When it comes to big data analytics, what is most important is this last component. More diverse sources of data are now accessible than ever before: organisations may obtain information from areas as diverse as loyalty card schemes, website interactions, CCTV cameras, reviews, app use data, and more. This data can all be separated into two categories: structured and unstructured.
Winning the data-centric digital business in this decade
Dell’s adaptive, secure, and resilient portfolio for the digital business
Structured data is what might immediately come to mind when you think of "data" — information neatly stored in a database or spreadsheet, for example.
Get the ITPro. daily newsletter
Receive our latest news, industry updates, featured resources and more. Sign up today to receive our FREE report on AI cyber crime & security - newly updated for 2024.
Unstructured data, in contrast, is the kind of information found in emails, phone calls, online interactions and other such seemingly opaque forms that defy easy analysis.
Big Data analytics programmes, such as Spark, Hadoop, NoSQL and MapReduce, can analyse both structured and unstructured data from a wide variety of sources, recognising significant patterns that can be used to drive new business proposals or adjust strategies.
Additionally, companies such as Google and Meta offer their own analytics insights, although these typically lack the raw insight offered by datasets sourced in-house.
Types of big data analytics
Diagnostic analytics
Put simply, diagnostic analytics answers the question “why did this happen?”. In big data analytics it can be a crucial antidote to anomalies in a data set, providing insight into the probability of why something turned out the way it did. In IT, this can be used to explain specific errors, shortcomings in a businesses’ systems, or even an unexpected success that an organisation wishes to repeat.
Descriptive analytics
If diagnostic analytics tells you why something happened, descriptive analytics can be considered a step back from this in that it simply tells a user what happened.
A simple example of descriptive analytics is data available through a central dashboard. This has been drawn together and aggregated by an analytical model to give an idea of how many times something has occurred, how many of something an organisation has in stock, or how much money has been spent in each department,
Predictive analytics
Predictive analytics is the bread and butter of big data analytics. It uses massive amounts of past data to build a statistical model for potential future outcomes. Businesses can use these in forecasting, and to help shape company strategy. It is also used as a cornerstone of AdTech, as it allows organisations to push advertising to consumers most likely to engage with it.
Prescriptive analytics
Prescriptive analytics leverages data sets to produce advice for IT decision makers. It could be considered a middle ground between diagnostic and predictive analytics, as it is capable of showing a series of outcomes for a business and give reasoning for why a particular path may be desirable over others.
To achieve this, it uses methods such as A/B testing and neural networks to build complex models that can produce expected outputs based on common patterns in data.
Trends in big data analytics
Tools to analyse data, whether it is in a data lake that stores data in its native format or a data warehouse, are still emerging. A number of different factors will determine how big data and associated analytics will operate in the future.
First is analytics in the cloud. As with a lot of things, big data analytics is increasingly hosted on the cloud. Hadoop can now process large datasets in the cloud, even though it was originally designed to do so on physical machine clusters. Among the companies offering Hadoop-based services in the cloud are IBM Cloud, Amazon's Redshift hosted by BI data warehouse, Google's BigQuery data analytics service and Kinesis data processing service.
Predictive analytics is also becoming more-commonly used. As technologies become more powerful, larger datasets will be able to undergo analysis and this, in turn, will increase the ability for change to be anticipated.
Automation: The key to optimised server management
Deliver modern digital end-user experiences, innovate with data, and more flexibly deliver IT services
Video analytics is also a good example of where big data is being both produced and deployed. Cloud-based CCTV systems extract billions of data points each day, and these are used to power facial recognition systems, manage crowd control at events and even aid smart town and city planning. Similar systems are also found in cameras and sensors used by driverless cars, many of which are used to improve this technology and make it safer for eventual use on real roads.
Finally, there's deep learning. This is a set of machine learning (ML) techniques that use neural networks to spot interesting patterns in massive quantities of binary and unstructured data, and infer relationships without needing explicit programming or models. This is critical for training artificial intelligence (AI), and right now is one of the most scrutinised areas of development in the tech sector.
The combination of Big Data and analytics is an important part of keeping organisations one step ahead of the competition, especially as cloud computing becomes a ubiquitous backbone for business. But firms must also foster the right conditions to enable data scientists and analysts to test theories based on the data that they have, in order to reap the most valuable results.
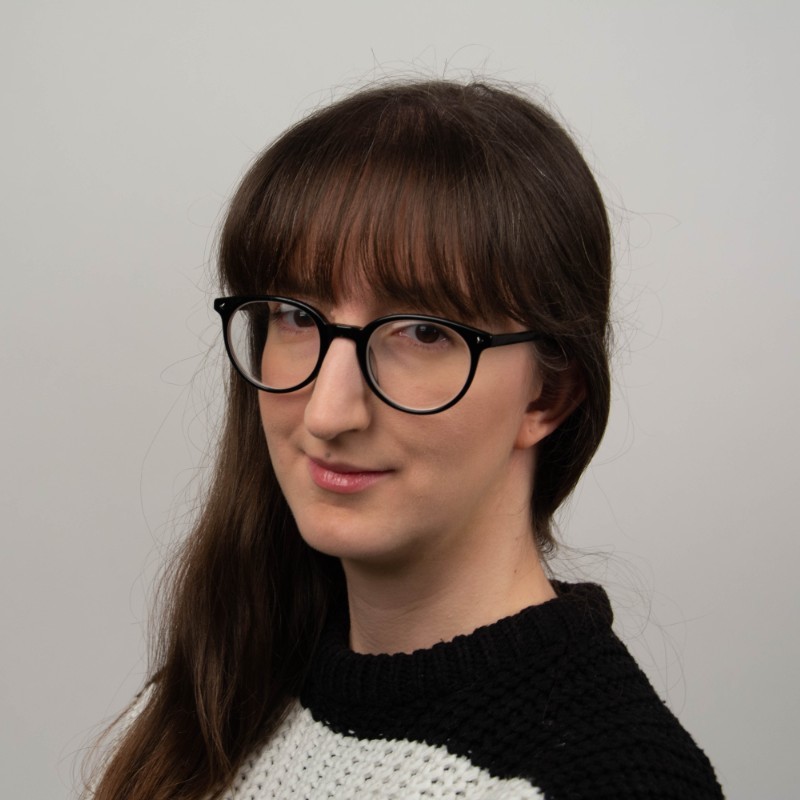
Jane McCallion is ITPro's deputy editor, specializing in cloud computing, cyber security, data centers and enterprise IT infrastructure. Before becoming Deputy Editor, she held the role of Features Editor, managing a pool of freelance and internal writers, while continuing to specialise in enterprise IT infrastructure, and business strategy.
Prior to joining ITPro, Jane was a freelance business journalist writing as both Jane McCallion and Jane Bordenave for titles such as European CEO, World Finance, and Business Excellence Magazine.