What good AI cyber security looks like today
Experts give their take on the role of AI in cyber crime and security, and reveal how businesses can protect themselves
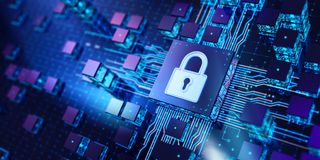
Artificial intelligence (AI) is revolutionizing cyber crime, and the security industry is playing catch-up. Defensive AI can help guard against offensive AI, but experts warn that AI crime is evolving so fast that even AI-enabled security software is not enough to stop it – and data-lockdown strategies such as 'zero trust' may be the only way forward.
When we explored the state of AI security in 2022, criminal gangs were busy embracing AI capabilities to trawl vast troves of data for insights accurate enough to help them devise video deepfakes, spear phishing and other targeted attacks that conventional security tools couldn't detect. In 2023, generative AI and machine learning (ML) have become established in cyber crime, and the tools of the trade are no longer limited to the dark web. Gangs use widely-available chatbots such as ChatGPT to automate the creation and distribution of personalized attacks on an industrial scale.
Security vendors have responded by ramping up the AI capabilities of their own tools, but business leaders are more fearful than ever. In a recent BlackBerry survey, a little more than half (51%) of IT decision-makers believed there'd be a successful cyberattack credited to ChatGPT by the end of 2023. At June's Yale CEO Summit, 42% said AI has the potential to destroy humanity within five to 10 years.
The dawn of autonomous AI malware
The biggest recent advance in AI is open source generative AI chatbots, based on natural language-learning models (LLMs), which anyone can use to create content. Criminals were quick off the blocks – weeks after ChatGPT was released for public use, software firm CircleCI was breached in an attack that used generative AI to create phishing emails and scan for vulnerabilities. By April
2023, Darktrace was reporting a 135% rise in novel social engineering attacks.
At the most basic level, attackers use LLM chatbots to simply gather information. "A chatbot could act as a mentor," IEEE member and professor of cyber security at Ulster University, Kevin Curran, tells ITPro. "There have been instances of AI chatbots being used to analyze smart contract code for any weaknesses or exploits."
Ambitious attackers, assisted by the growing trade in AI hackers for hire, have used generative AI to refine phishing operations, says Freeform Dynamics analyst Tony Lock. "They're using chatbots to generate voice sounds and fake imagery, and to copy people's published writing styles. This has all become much more sophisticated and industrialized in 2023."
Martin Rehak, CEO of security firm Resistant AI and a lecturer at Prague University, says we are already seeing AI-enabled criminals exploiting organizations' AI-based security systems. "Rather than attacking the security systems, criminals are attacking the automation and AI systems companies rely upon to conduct business online."
Get the ITPro. daily newsletter
Receive our latest news, industry updates, featured resources and more. Sign up today to receive our FREE report on AI cyber crime & security - newly updated for 2024.
The potential for generative AI to do all this autonomously hasn't gone unnoticed by the AI industry. None other than Sam Altman, CEO of ChatGPT developer OpenAI, admits he's "worried" about the ability of chatbots to distribute large-scale disinformation and write malicious code, potentially by themselves.
The message from the security industry is that to face down AI threats, you need AI defenses. Brands from CrowdStrike to Google have put AI at the center of their enterprise security systems, and a Freeform Dynamics survey of 50 CIOs found that, of all the vendors encountered by respondents, nearly all (82%) claimed their products used AI.
Accordingly, the global AI security market grew from $13.29 billion in 2021 to $16.52 billion in 2022, and is forecast by SkyQuest to reach $94.14 billion by 2030.
Unlike conventional endpoint security, AI can detect the tiniest potential risk before it enters a system. For example, Microsoft Azure's secure research environment uses smart automation to supervise the user's business data, with ML ready to leap into action if an anomaly is detected. Response times are continually slashed as the algorithms learn from their own experiences and from other organizations, via samples shared in the cloud.
"AI tools can organize information on a global scale," says Carlos Morales, SVP of solutions at cloud security firm Vercara. "They can draw correlations between data from different defense solutions, and detect and act on new attacks proactively."
Many AI security services now include elements of deep learning and neural networks, which are effectively artificial brains that learn to automatically and instantly "know" the difference between benign and malicious activity. Deep learning can autonomously detect network intrusions, spot unauthorized access attempts, and highlight unusual behavior that may indicate an attack is afoot, often in near- real time.
AI can also now help limit the damage an attack can do. "It triggers set defense procedures in response to an incident," says Simon Bain, CEO of security platform OmniIndex. "If a specific database or blockchain node is attacked, AI can isolate the damage to that single location and stop it spreading into other areas."
The ability of generative AI to generate advice from huge amounts of chaotic data very quickly also makes it useful to defenders. A security chatbot can inspect a system's security controls and configurations, pointing out gaps and recommending policies. The next step in this rapid evolution will be for AI systems to autonomously audit, assess and validate our security controls.
Security teams have already used generative AI to build models that help them detect malware written by known agents, predict what it will do next, and swing into action automatically when an attack or variant is detected.
Firms including Darktrace have developed smart attack simulations that will autonomously anticipate and block the actions of even the most inventive AI-powered hacker.
"Proactive security and simulations will be incredibly powerful," says Max Heinemeyer, VP of cyber innovation at Darktrace. "This will turn the tables on bad actors, giving security teams ways to future-proof their organizations against unknown and AI-driven threats."
Inevitably, security vendors are confident about the AI capabilities of their products. But business leaders remain wary, fearing a level of hype about AI in security products. More than half (54%) of Freeform Dynamics' respondents said they're "cautious" or "suspicious" of vendors' claims about their AI capabilities.
Whether or not this suspicion is warranted, businesses are wise to resist seeing AI-enabled security software as a magic bullet, says Curran.
"Like other technical innovations, AI has a tendency to be over-hyped, especially in cyber security," says Curran. "But it does have the potential to significantly impact and influence the way organizations protect themselves in the coming years."
The criminal exploitation of generative AI extends far beyond writing emails and code. Most LLM chatbots are free to use and exist outside an organization's tech stack, so security teams have no control over them – and they are replete with data leak risks.
Is zero trust the best defense?
Imagine you’re a product manager drawing up a product proposal, and you use an LLM, say ChatGPT, to check over your document for readability and clarity. This exchange will likely involve highly confidential business details. This example is far from just theoretical, however. In July, Deloitte reported 4 million UK workers using generative AI tools for work, and this number will keep growing.
Data leaks and generative AI are a reality we must already contend with. In March, a glitch exposed users’ chat histories and titles on ChatGPT’s sidebar. OpenAI supposedly patched the bugs, but despite this Apple banned ChatGPT for all employees as an extra security precaution. Security specialists Group-IB, discovered stolen ChatGPT credentials in over 100,000 malware logs, in June.
Security solutions leverage AI to prevent the use of stolen credentials by threat actors. For instance, Human Security’s offering aims to identify and block compromised credentials on platforms in real-time. However, many experts, including analysts at Forrester and Google Cloud, believe the sole effective defense against attacks like these is to implement a zero trust security model. A zero trust approach treats all data as untrustworthy and enforces rigorous access controls across all devices, including personal phones.
Notably, of the UK-based experts we spoke to for this article, none specifically advocated zero trust, which Gartner suggests has stronger adoption rates in the US compared to privacy-focused UK. Most analysts stressed the importance of improved employee training on data sharing and verification, especially for remote work.
"The best defense against AI-generated phishing is a strong policy," says Ron Culler, VP of cyber development programs at professional IT body CompTIA. "Take spear phishing, where a bad actor attempts to steal credential information. An organization can safeguard against these attempted attacks by implementing dual authentication policies that require a second person to verify requests, or require the use of PIN codes to verify identity."
The limitations of AI security
Just as driverless cars are set to transform transport, autonomous AI security systems may one day render human supervision unnecessary. Businesses can now
use AI and ML to help fill the skills gap, and minimize the types of human mistakes that lead to massive security flaws. For example, the new AI Navigator 'copilot' tool from D2iQ intelligently detects problems such as poor security configuration.
But as we approach 2024, human input is still crucial to any AI-enhanced defense strategy. Generative AI trained on bad data can deliver poor decisions and inaccurate information which, if unchecked, may do more harm than good to a business's security. It's telling that, like many AI-enabled systems, AI Navigator comes with 24/7 human support teams.
"These systems are good enough at separating the wheat from the chaff as far as security indicators are concerned, but we still need human incident responders to investigate the remaining highlights," says Corey Nachreiner, CSO of WatchGuard. "They can also make very bad decisions – hallucinations, as they're called – if the data they pull from is inaccurate or bad. They are not actually cogitating."
"We have to be realistic about AI's limitations," says Mark Stockley, cyber security evangelist at Malwarebytes. "It can potentially lighten the load, but there will be a role for specialized human threat hunters for a while yet."
Jane Hoskyn has been a journalist for over 25 years, with bylines in Men's Health, the Mail on Sunday, BBC Radio and more. In between freelancing, her roles have included features editor for Computeractive and technology editor for Broadcast, and she was named IPC Media Commissioning Editor of the Year for her work at Web User. Today, she specialises in writing features about user experience (UX), security and accessibility in B2B and consumer tech. You can follow Jane's personal Twitter account at @janeskyn.