11 best job boards for tech
The best places to apply for tech jobs online, and the tips you need to do so successfully
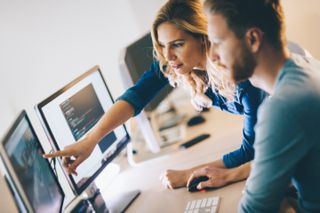
Searching for a new job can be a daunting and challenging experience at the best of times, let alone during the current economic climate.
In fact, 2020 was one of the most difficult years for finding employment, especially for those just starting out in tech, with most companies, from Salesforce and Mozilla to LinkedIn and Monzo, opting for massive redundancies as a result of the global pandemic.
However, it looks like the job market could be recovering. Ericsson has vowed to create 800 direct and indirect employment opportunities as part of its 5G network rollout plan, including jobs in build engineering, project management and field operations, and Salesforce, which cut around 1,000 jobs in August 2020, announced a month later that it would be adding 12,000 jobs over the course of 2021.
That is why it’s important to not lose hope – after all, with a combination of perseverance and luck, you might just be able to score your dream job.
Thankfully, job boards are here to make it an easier process for everyone – employer and candidate alike. And although plenty of job-seeking websites, including Indeed and LinkedIn, focus on open positions across all industries, there exist some which are specifically tailored to the tech sector, such as Crunchboard or Tech Ladies.
Here we compiled some of the best job boards with a focus on the tech industry, and added some useful tips on how to navigate them with ease.
11 best tech job boards
These 14 job boards crush in for tech jobs. Whether its the sheer volume of jobs or the ability to sort the listings to fit you, these are the best of the best.
Get the ITPro. daily newsletter
Receive our latest news, industry updates, featured resources and more. Sign up today to receive our FREE report on AI cyber crime & security - newly updated for 2024.
1. Indeed
You've undoubtedly heard of Indeed, which is one of the best-known job boards with 250 million unique visitors every month. While it isn't solely focused on tech, Indeed adds 10 jobs per second worldwide and it's easy to search for keywords, job titles, and companies that you're interested in.
The job board also offers a wealth of resources, so users can get things like tips for negotiating salary or how to detect common job scams while they're on the hunt.
2. LinkedIn
LinkedIn’s Jobs section is another great stop in your search for a job in tech.
You can filter the databasae by title, skill, or company, and now it even offers a feature you can turn on to only see remote jobs. LinkedIn, while a general job database like Indeed, is popular enough to provide you with plenty of tech jobs to apply to. If you’re a member of LinkedIn Premium, it will also show you how you compare to other candidates.
3. Tech Ladies
Tech Ladies is specifically for women in tech and requires users to apply for membership. However, membership is free and comes with a subscription to a weekly insiders' newsletter, so don't let the membership application ward you off. Tech Ladies screens not only applicants but job posters, making sure that users don't have to deal with toxic interviews or scams.
It’s also worth mentioning that Tech Ladies has more than 100,000 members, making it more than just a job board. As it states on its home page, "Above all else, we are a community." The site regularly schedules webinars for women working in tech and offers coding classes, career coaching, and more - plus it's a great way to network with other women in the field.
4. GeekWork
If you’re looking for a job in tech and have an interest in working for a startup, head over to GeekWork.
GeekWork allows users to filter jobs by tags, limiting searches to machine learning, social media, Linux, Python and more. The job board also segments its jobs by consulting, freelance, part-time, full-time and temporary opportunities.
5. DataJobs
For data scientists who need a new job, there is no shortage of tech jobs on DataJobs. Founded by Frank Lo, Director of Data Science at Wayfair, DataJobs is a place where data specialists can browse open jobs to find the right fit at top companies.
6. AngelList
AngelList calls itself the “world’s largest startup community.” By uploading your resume to AngelList Talent you can apply to over 130,000 tech jobs with one application.
Twitch, Stripe, Instacart, Uber and Discord have each posted open positions to AngelList too.
7. CrunchBoard
If you’re familiar with TechCrunch then you may know about CrunchBoard.
For jobs in tech, CrunchBoard is a great resource. Search its database by keyword or sign up for job alerts to keep up to date on the latest jobs being posted to this tech board.
8. Mashable Job Board
Use the Mashable Job Board to find jobs in tech, engineering, web development and more.
Unlike other tech job boards, Mashable Job Board also allows users to search its database using its company directory.
9. WhiteTruffle
WhiteTruffle is less of a job board and more of a matchmaking service.
Create a profile, and WhiteTruffle will match you to companies seeking employees with your experience and skill set. Once it makes a match, WhiteTruffle will introduce you to the company, coordinate interviews and send job offers right to your inbox.
With 7,500 tech companies using its services, there’s no reason not to give WhiteTruffle a shot when looking for a job in tech.
10. IT Job Pro
Nine traits you need to succeed as a cyber security leader
What characteristics and certifications make a successful cyber security leader?
According to Entrepreneur Magazine, IT Job Pro is the most popular niche site for tech jobs.
Founded in 2010, over 2 million job seekers have used this job board. Check out its current listings, and you’ll find open positions at companies like Tesla, Teradata, pony.ai and more.
11. Hackernoon
For hackers in search of open jobs in tech, consider heading over to Hackernoon to kickstart your job hunt.
Search Hackernoon’s extensive database by keyword, category and location to find the job best suited for your needs.
Tips for navigating online job boards
Once you find an amazing tech job board that suits you, you may still find it tough to navigate the site and find the job you want. Here are a few tips to help navigate these tech job boards.
1. Update your resume
Before starting your job hunt, examine your resume and LinkedIn profile. They will serve as a first impression when applying for your next job. Use your resume to highlight your work history, greatest accomplishments and most valuable skills.
When it comes to LinkedIn, consider asking coworkers or former managers to provide you with a recommendation. Above all, make sure your resume and LinkedIn profile are concise, easy to read and grammatically correct.
Keep in mind, the same advice applies when writing a cover letter.
2. Knowing how to use jobs boards
You probably know the basics of how to use a job board, but that knowledge is always worth refreshing.
Before diving deep into a job board’s database, familiarize yourself with how to navigate it. For instance, while some boards allow you to search for job openings using specific keywords, others allow users to search for openings by company.
3. Look at active job postings
When it comes to applying for tech jobs online, do your due diligence by narrowing your search by the date the position was posted. For the most up-to-date job postings, consider keeping the date range to 30 days.
Old job postings may be interesting to look at, but it’s hard to tell if those jobs are still active, as some job boards don’t require companies to take down posts after filling a job.