The best big data technologies
We round up the top big data storage, data mining, analysis and visualisation tools
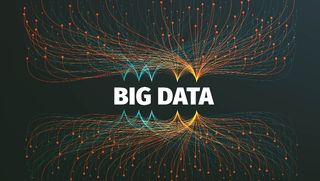
The technology driving the modern world of business and digital transformation is artificial intelligence (AI). But what fuels AI is something more complex and diverse, and it's something that is constantly generated and exceedingly valuable: data.
Data is essential information; personal or customer details, habits and traits, likes and dislikes. In business terms, it's about how we interact with companies online and what that tells the company about us. That is how basic targeted advertising works - you have looked at companies product, that product will then be seen on Google Ads or in YouTube ads before the video you actually want.
With so many of us online, and so many businesses getting our details, we are now in the age of ‘big data’, which is at the heart of digital transformation and also the EU's groundbreaking GDPR legislation. While the information is being generated all the time, it isn't free for all and is subject to laws and regulations. Businesses that violate these can be in for some heavy fines.
Aside from its legality, big data already present businesses with challenges. Namely, the sheer size of data one can extract and use is too big for the humans to analyse for insights. This is where machine learning and artificial intelligence is coming of age. The ability to process data in real-time and extract insights for instant use is the key to digital transformation. At best, it's a super tool for employees to enhance their workflows, but at worst, it's a powerful fuel for automation that may one day replace us.
Data storage
Cloud-centric storage tools are key to ensuring you’re able to store the largest possible amount of data, with various options available to allow your organisation to keep data in a secure and accessible way.
Hadoop
This is an open source platform which normally stores massive datasets through clusters. Hadoop supports both structured and unstructured data and scales without any hassle, making it a great option for companies likely to need extra capacity at short notice. This platform can also handle a huge number of tasks without latency. Overall, it's a great option for organisations with the developer resource to implement Java, although it does demand effort to establish.
Get the ITPro. daily newsletter
Receive our latest news, industry updates, featured resources and more. Sign up today to receive our FREE report on AI cyber crime & security - newly updated for 2024.
MongoDB
MongoDB is very useful for organisations that use a combination of semi-structured and unstructured data. This could be, for example, organisations that develop mobile apps that need to store data relating to product catalogues, or data used for real-time personalisation.
RainStor
Rather than simply storing big data, Rainstor compresses and de-duplicates data, providing storage savings of up to 40:1. It doesn't lose any of the datasets in the process, making it a great option if an organisation wants to take advantage of storage savings. Rainstor is available natively for Hadoop and uses SQL to manage data.
Data mining
Once you have your data stored, you'll need to invest in tools to help you find the information you want to analyse or visualise. Our top three tools will help you extract the data you need without the hassle of manually trawling through it all (a task that's impossible for humans to do anyway if you hold thousands or more records).
IBM SPSS Modeler
IBM's SPSS Modeler can be used to build predictive models using its visual interface rather than via programming. It covers text analytics, entity analytics, decision management and optimisation and allows for the mining of both structured and unstructured data across an entire dataset.
KNIME
KNIME is a scalable open source solution with more than 1,000 modules to help data scientists mine for new insights, make predictions, and uncover key points from data. Text files, databases, documents, images, networks and even Hadoop-based data can all be read, making it a perfect solution if the data types are mixed. It features a huge range of algorithms and community contributions to offer a full suite of data mining and analysis tools.
RapidMiner
RapidMiner is an open source data mining tool that allows customers to use templates rather than having to write code. This makes it an attractive option for organisations without a specific resource or if they're just looking for a tool to start mining data. A free version is also available, although it's limited to 1 logical processor and 10,000 data rows. The tool also provides environments for machine learning, text mining, predictive analytics and business analytics to help with the entire process.
Data analysis
Got the data you need? Now it's time to find the most powerful tools to help you analyse it in order to glean key insights into your business, your customers or the wider world. Here, we round up our favourite data analysis tools.
Apache Spark
Apache Spark is perhaps one of the most well-known big data analysis tools, built with big data at the forefront of eveything it does. It's open source, effective, and works with all major big data languages including Java, Scala, Python, R, and SQL. It's also one of the most widely used data analysis tools and is used by all-sized companies, from small businesses, to public sector organisations and tech giants like Apple, Facebook, IBM, and Microsoft.
Apache Spark takes analysis one step further, allowing developers to use large-scale SQL, batch processing, stream processing, and machine learning in one place, alongside graph procession too. It's super-flexible too, running on Hadoop (for which it was originally developed), Apache Mesos, Kubernetes, by itself as a standalone platform, or in the cloud, making it suitable for businesses of all sizes and in all sectors.
Presto
Like Apache Spark, Presto is an open source tool, using distributed SQL queries, designed to run queries against data as a powerful interactive analytics engine. It suports both non-relational sources, such as the Hadoop Distributed File System (HDFS), Amazon S3, Cassandra, MongoDB and HBase, plus relational data sources such as MySQL, PostgreSQL, Amazon Redshift, Microsoft SQL Server, and Teradata, making it a useful tool for businesses operating both types of database.
It's also used by huge corporations such as Facebook. In fact, the scial network was a major contributor to its development, although Netflix, Airbnb and Groupon were also involved in its development to make it one of the most powerful data analysis tools around.
SAP HANA
Data analytics is just one aspect of SAP's HANA platform, but it's a feature it does exceptionally well. Supporting text, spatial, graph and series data from one place, SAP HANA integrates with Hadoop, R and SAS to help businesses make fast decisions based on invaluable data insights.
Tableau
Now owned by Salesforce, Tableau combines data analysis and visualisation tools and can be used on a desktop, via a server or online. The online version has a big focus on collaboration, meaning you can easily share your discoveries with anyone else in your organisation. Interactive visualisations make it easy for everyone to make sense of the information and with Tableau Cloud's fully hosted option, you won't need any resource to configure servers, manage software upgrades, or scale hardware capacity.
Splunk Hunk
Designed to run on top of Apaches Hadoop framework, Splunk's Hunk is a fully-equipped data analytics tool which can generate graphs and visual representations of the data it is fed, all manageable through a dashboard. Queries can be made against raw data through Hunk's interface, while graphs, charts and dashboards can be quickly created and shared through Hunk's interface. It also works on other databases and stores as well, including Amazon EMR, Cloudera CDH, and Hotronworks Data Platform among others.
Data Visualisation
Not everyone is adept at taking key insights from a list of data points or understanding what they mean. The best way to present your data is by turning it into data visualisations so everyone can understand what it means. Here are our top data visualisation tools.
Plotly
Plotly supports the creation of charts, presentations and dashboards from data analysed using JavaScript, Python, R, Matlab, Jupyter or Excel. A huge visualisation library and online chart creation tool makes it super-simple to create great looking graphics using a highly effective import and analysis GUI.
DataHero
DataHero is a simple to use visualisation tool, which can suck data from a variety of cloud services and inject them into charts and dashboards that make it easier for the entire business to understand insights. Because no coding is required, it's suitable for use by organisations without data scientists in residence.
QlikView
With a suite of capabilities on offer, QlikView allows its users to create data visualisations from all manner of data sources with self-service tools that remove the need for complex data models to be in place. Straightforward visualisation is served up by QlikView running on top of the company's own analytics platform, which can be shared with others so decision made upon trends the data revealed can be collaborative.
More advanced capabilities allow for QilkView's visual analytics to be embedded into apps, while dashboards can guide people through the production of analytics reports without needed them to have an understanding of data science.
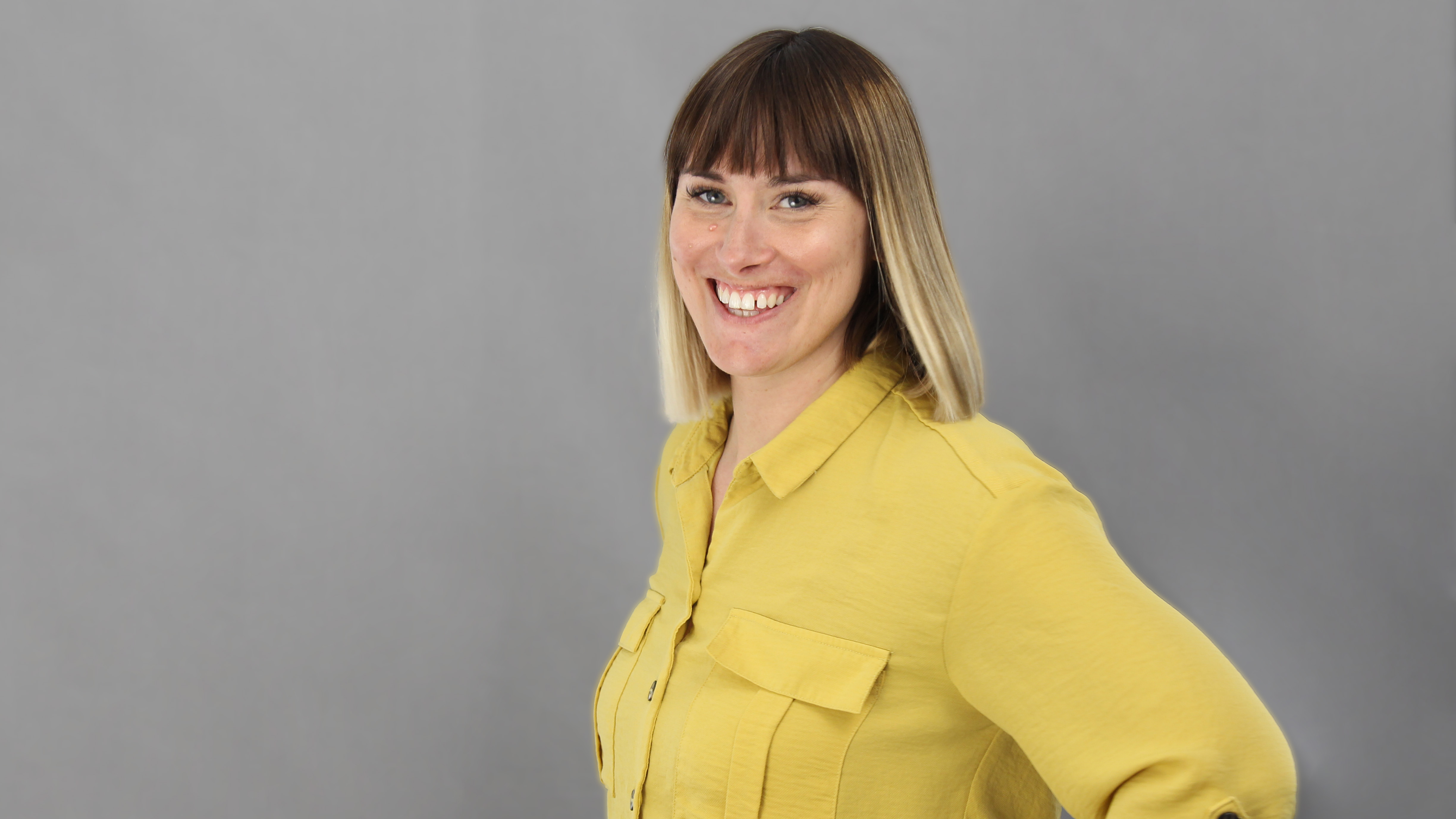
Clare is the founder of Blue Cactus Digital, a digital marketing company that helps ethical and sustainability-focused businesses grow their customer base.
Prior to becoming a marketer, Clare was a journalist, working at a range of mobile device-focused outlets including Know Your Mobile before moving into freelance life.
As a freelance writer, she drew on her expertise in mobility to write features and guides for ITPro, as well as regularly writing news stories on a wide range of topics.