Three keys to successful data management
Companies need to take a fresh look at data management to realise its true value
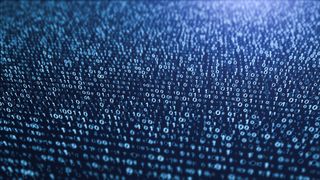
Managing data within an organisation can involve a large number of processes, people, and policies. The more data a company produces, collates, and holds across multiple systems, the more important it is to have a robust data management strategy in place, get the most value out of it, and make the most informed business decisions.
Why smart businesses view a data fabric as an inevitable approach to becoming data driven
Adopting a data-driven strategy for success
Data creation during the pandemic reached an all time high and, according to Statista, data – in particular data storage – is set to grow at an annual rate of around 20% over the next three years. Now with this increase being the driving force behind the majority of the world becoming digitised, a data management platform that can process and store large volumes is fundamental.
Whether you've decided to manage data in-house, or outsource it to a data management service, technology can eliminate many manual management tasks, which in turn can reduce the chance for errors. It can also limit data quality issues by incorporating a combination of functions to ensure data is accurate, accessible, and most importantly, secure.
In adopting best-in-practice data management, organisations need to cover various steps including data processing, storage, governance, formatting, and analysis, and build a foundation on which all data technology will sit.
And as part of this digital transformation, companies should include a fresh look at enterprise data management, to address questions around storing stale data, and ensure they have the most valuable data insights available.
Achieving this requires addressing three key capabilities:
Optimised data access
Enterprise data management should ensure users have seamless, secure access to whatever data they need, no matter where it may be physically stored. It also needs policy-based contextual awareness to deal with data across its lifecycle, so you're no longer storing data that no one needs.
Get the ITPro. daily newsletter
Receive our latest news, industry updates, featured resources and more. Sign up today to receive our FREE report on AI cyber crime & security - newly updated for 2024.
Data visibility
Global data visibility and control of data is another crucial element, and it must be independent of any application or storage location. That means data should be visible regardless of whether it's stored on an in-house storage array or associated with a cloud-based application. There should also be absolute clarity on who has access to and ownership of all data to manage risk.
Service durability
Why smart businesses view a data fabric as an inevitable approach to becoming data driven
Adopting a data-driven strategy for success
Enterprises need to ensure data is always protected and available by making the end-to-end service infrastructure across their different landscapes resilient, along with making sure this infrastructure can quickly rebound from any service disruption.
The unprecedented growth in data demands that organisations take a fresh look at enterprise data management. It's imperative not only to get costs under control, but to provide the sort of data visibility, resiliency, protection and optimised access that's required if enterprises are to make effective use of their data.
Esther is a freelance media analyst, podcaster, and one-third of Media Voices. She has previously worked as a content marketing lead for Dennis Publishing and the Media Briefing. She writes frequently on topics such as subscriptions and tech developments for industry sites such as Digital Content Next and What’s New in Publishing. She is co-founder of the Publisher Podcast Awards and Publisher Podcast Summit; the first conference and awards dedicated to celebrating and elevating publisher podcasts.