How to improve database costs, performance and value
We look at some top tips to get more out of your databases
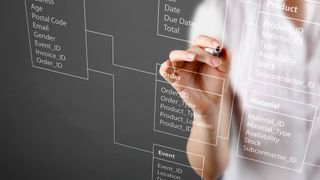
While databases are one of the foundational building blocks of enterprise IT, many organisations may find themselves blindsided by the difficulty of running them effectively. Poorly-optimised databases can become serious time sinks, incurring unnecessary costs and distracting IT teams from other important tasks.
Luckily for database administrators (DBAs), there are various tricks and techniques that can be employed to improve and speed up database management and create more efficient and cost-effective systems. Here are our top tips.
Automate management tasks
DBAs’ duties cover an enormous range of tasks, including everything from backup and recovery jobs to storage management and configuration – many of which are highly mundane and repetitive. This makes them ripe for automation. By automating these simple tasks, DBAs can free up their days for more rewarding, interesting and innovative activities that are a better use of their valuable time.
There are many options when it comes to automating database management tasks. Many DBAs will choose to write their own custom tools crafted to their specific needs, while others may rely on the automation tools that database management software vendors have built directly into their products. Whichever way you choose to go, automating elements of your database management can help you save both time and money, as well as reducing recovery time in the event of any failures.
Deploy containers and virtual machines
Virtualisation and multi-tenancy are nothing new in the worlds of enterprise IT and software development, but they can have big advantages for database management. If you’re running your databases on single-tenant servers, then porting them to VMs could bring big savings. For starters, this allows you to host multiple databases on the same infrastructure, which can cut down on licensing and hardware investment costs. It also offers more flexibility in porting, replicating and modifying databases, although some may find vertical scaling becomes challenging.
If you want even more portability, you may wish to consider containerising your databases. This works well as part of a DevOps environment and integrates nicely with both CI/CD pipelines and multi-cloud architectures. The lightweight nature of containers means you can run large amounts on a single server, and they also lend themselves to high levels of automation which (as we covered above) can have big benefits for cost and efficiency.
Optimise SQL queries
Not all database queries are created equal. In fact, inefficient or poorly structured SQL queries often take longer to run, and when run on live production databases they can introduce errors and cause outages for other database users. By putting in a little more legwork in fine-tuning their initial queries, database operators can speed up their operations to improve the long-term health and performance of their databases.
Get the ITPro. daily newsletter
Receive our latest news, industry updates, featured resources and more. Sign up today to receive our FREE report on AI cyber crime & security - newly updated for 2024.
For example, when selecting fields as part of a query, it can be tempting to use SELECT* to quickly select all records, or using SELECT DISTINCT to identify unique results. However, this can lead to unnecessary processing if it’s being run on large databases. Instead, thinking about the results you’re looking for and structuring your query around those specific fields will cut down on the processing power required to run it. Similarly, using INNER JOINs instead of Cartesian Joins made using WHERE clauses can massively reduce the amount of work being done by the system.
Manage database growth
One of the biggest factors that affect both the performance and speed of a database is how large it is. The bigger it is, the longer it takes to search through it and deliver results from a query. Size also plays a factor in process and transaction costs for cloud-hosted databases, or in hardware upgrade cadences for on-premise systems.
The best way to ensure that your database doesn’t expand at an unsustainable rate is to set up your schema and validation rules so that it contains only data which is going to be necessary to its operation. Storing large amounts of infrequently or partially used records can increase the cost and the time it takes to run queries.
Retire old or unnecessary data
As we’ve just covered, keeping your database streamlined is a key part of making sure they remain efficient and cost-effective. However, even if you’re restricting database inputs to the bare minimum of fields that need to be there, the size of your database will inevitably swell over time. If you’re finding that your database is getting a bit unwieldy, it might be worth seeing if you can streamline it by removing old entries that no longer need to be in there.
The specifics of what records can safely be deleted and when will depend on the specific purpose of your database, but setting clear data deletion policies can help keep its size to a manageable level. Another added bonus is that this can ensure that your queries aren’t returning as many irrelevant junk results, and may even help with GDPR compliance in the case of databases containing personal information. Large tables can also be partitioned to help stop them from getting too big, and indexes should be monitored to ensure they're still being used; any that aren't can be removed to free up additional space.
Time your queries
Databases have many advantages, including that many of them can be used by a number of different applications and users for different things, and that many are multi-purpose. Despite this, it is necessary to think about the impact queries can have on performance for the rest of the business. If you’re running large, complex queries, for example, that need lots of processing power, it may cause other applications and queries to run slower.
Are you planning to run any particularly heavyweight queries? It may be worth considering how time-sensitive they are as if it isn’t urgent, it might be worth organising them to run outside of peak business hours when there will probably be less activity on the database. This helps to reduce the impact on the database’s efficiency and keep disruption to a minimum.
Perform regular audits
At the end of the day, it’s important to remember that none of the tips detailed above will work well if you don’t perform regular audits on your databases. Just like humans need health checkups, databases also need to be thoroughly inspected from time to time in order to ensure that everything is running like clockwork. As your organisation grows and evolves over time, so does the usage and purpose of its databases. Therefore, these regular audits should include monitoring elements such as input rules, data deletion policies, schema, and usage patterns, in order to ensure that your databases meet your business’ needs.
Although it might seem cumbersome and time consuming to review an entire database every few months, it does benefit the company in the long run. With regular audits, you can easily identify which areas should be optimised in order to save running costs, streamline tasks, and what actions to avoid in order to minimise mistakes as well as potential outside threats, such as data breaches or thefts.
Last but not least, make sure your database logs are compliant with data protection regulations such as the EU’s GDPR or the UK’s Data Protection Act 2018, especially if your organisation deals with personal data. Regular audits mean that you will be able to keep an eye on changing legislations and ensure that your organisation’s databases follow the rules, while also letting it reap the benefits of data storage. After all, data is nowadays crucial to delivering the highest level of services, therefore it’s important that your databases are kept to the same standards.
Adam Shepherd has been a technology journalist since 2015, covering everything from cloud storage and security, to smartphones and servers. Over the course of his career, he’s seen the spread of 5G, the growing ubiquity of wireless devices, and the start of the connected revolution. He’s also been to more trade shows and technology conferences than he cares to count.
Adam is an avid follower of the latest hardware innovations, and he is never happier than when tinkering with complex network configurations, or exploring a new Linux distro. He was also previously a co-host on the ITPro Podcast, where he was often found ranting about his love of strange gadgets, his disdain for Windows Mobile, and everything in between.
You can find Adam tweeting about enterprise technology (or more often bad jokes) @AdamShepherUK.