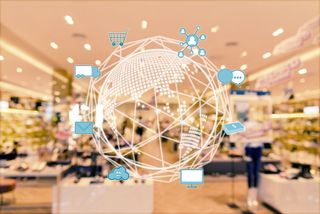
The last two decades have seen upheaval on the high street, and consequently, the retail industry has faced huge challenges. As the industry reconfigures itself, accelerated by the coronavirus pandemic, technology and data have a huge part to play in its transformation.
While e-commerce platforms and home deliveries might be the first things that spring to mind when you think about digital retail, this is just the tip of the iceberg. Emerging technologies such as robotic process automation, AR and 5G will revolutionise retail spaces and in-store customer experiences as much as online shopping.
Digitally-enhanced store experiences
Even before the events of 2020, a transformation of the high street was well on its way. With consumers increasingly choosing ‘clicks over bricks’, many retailers are rethinking their store space. For some, having vast amounts of space simply isn’t a good investment, and this is especially true if retailers don’t take changing consumer habits – such as the rise of contactless payment to the popularity of services like click and collect – into account.
Does this mean the end of the high street shop? Not necessarily, but it does mean a dramatic transformation of the industry – especially now that COVID-19 is set to propel demand for contactless environments. We’ve already seen the widespread replacement of traditional tills with self-service checkouts, but now we may see these disappear altogether – replaced by pay-as-you-go phone apps. Daren Ward, executive partner at Reply, predicts that “till banks will start to disappear, and of course that frees up two things – it frees up shop floor space, so that has an influence on how shops start to operate, but it also frees up time and people. People sitting behind the till will be able to walk around the shop floor and actually engage with customers.”
This engagement is crucial for retail spaces to survive in the modern world, and Ward believes retail apps are vital. He explains: “The key trend is going to be around how we engage with customers. We've seen in other regions around the world a big uptick in the use of apps. So if you think about that whole wallet experience, the loyalty experience, the contactless experience, it can be improved greatly if you wrap it around an app. And can start to build a very different experience in the stores.”
Using customer data, retailers will be able to tailor offerings and experiences to individuals, “we will start to personalise the journey in the store, much as we do online,” Ward explains. For example, much like targeted online ads, fashion retailers can work out an individual's style preferences, suggesting alternative products to those on display as they walk around the store. And, with a single branded app, retailers could utilise emerging technologies such as AR, VR and AI to transform and personalise the customer experience. The sophistication of these exciting technologies combined with the promise of 5G to support them means the potential for apps is significant.
Beyond the traditional store
Taking the technology a step further, retail spaces may no longer be packed full of products for customers to browse. Much like the Argos model, we’ll likely see more retailers opting to create small, smart technology-equipped shop fronts to order goods from connected warehouses. “The biggest problem that we see in retail today is cost, and managing costs, as margins are shrinking rapidly,” Ward explains. Therefore, it’s vital that retailers use the space they have effectively – whether that’s using it as more of a warehouse, or collaborating with other companies to share retail space.
The popularity of home deliveries and services such as ‘click and collect’ are also pointing towards this shift. With automation, retailers can gain a much better understanding of what stock they have, where it is, and the logistics of getting it from storage to the customer. “We've done some work with a few of our clients on ‘ship from store’,” says Ward. With stores often very conveniently located, it makes sense for retailers to take the customer’s location into account when shipping an item, “rather than sending it from a warehouse up in the Midlands, say there's a store only two miles around the corner. If I can hold some stock in that location, then actually my distribution becomes relatively easy.”
To stay competitive, retailers also need to understand how their various channels work together. Treating your e-commerce platform as a completely separate entity to your physical store is no longer a viable business model, neither is ignoring the potential of social media to engage customers. “It's not about the channel, it's about the conversation,” explains Ward. “The channels are made by retailers, they're not made by customers, and they don't really care about channels, they just want to interact with the brand.” However and wherever a customer interacts with your brand, you should know who they are and what they need.
This may mean combining the at-home and in-store experience, utilising space and the skills of your employees. For example, Reply has been working on a video chat capability which can perform a skills search and connect the customer with an expert, rather than simply whoever picks up the phone at a call centre. Ward says: “A classic example, one that we've done overseas, is with DIY. So if you're building a patio, you can have an expert in the store on the phone with you looking at what you've done so far; what tools you've got, what you're missing, and really help orchestrate that experience for you.”
Using data and technology appropriately
Retailers have been gathering customer data for years, but many simply don’t know what to do with it. There’s a fine line between utilising that data in a way that’s useful to the retailer and customer without irritating that customer. And the simple solution is relevance – ensuring that the way you communicate with your customers is appropriate to them.
Targeted ads are an obvious example of this. If someone has bought a toaster, it’s probably because their old one is broken, not because they collect toasters – and if they continue to get bombarded with ads for toasters on every website they visit they are soon going to lose faith in your brand. Ward explains: “Everybody talks about ‘well, what are we going to give back to the customer if we use their data?’ They immediately think of points and loyalties but actually they should just be relevant. That's quite a bonus for most customers.”
The same is true when deciding which technologies to utilise. To stay competitive, retailers must embrace digital transformation, but it’s also essential that innovation is relevant to business objectives. Ward urges retailers: “Innovation is important, but please make it appropriate for your brand. We see some companies who are still talking about things like magic mirrors. And of course, where does the magic mirror go? Well, it goes into the changing room. Do you really want your customers in the changing room? If you've got a value proposition and it's all about turnover, you probably don't want people dwelling in your changing rooms. So pick innovation appropriate to your brand.”
There is still a long way to go to educate retailers on the technology available to them and the best ways to utilise it. As with the magic mirror example, many companies get excited by new technologies and want to use them wherever they can, even if it’s not appropriate. On the other end of the spectrum, you have businesses reluctant to embrace innovative technologies. For example, Ward has been surprised by the slow uptake of automation, suggesting this may be because organisations associate it with specific tasks, usually in areas such as HR or finance.
Part of Reply’s work is educating their clients on the ways that technologies like process automation can be used across the business, sometimes all the way up the supply chain. "The automation doesn't have to be customer facing to improve customer experience," Ward notes. The key is not just to remove costs, but deliver a better experience. “If you automate a process, you should also seek to improve the customer experience at the same time, those two things can and should go hand in hand.”
Reply is working with customers on projects from “last mile” solutions to managing deliveries to 3D virtual interactive showrooms. The future for retail is exciting and full of endless possibilities – as long as customer experience is at the heart of it.
Get the ITPro. daily newsletter
Receive our latest news, industry updates, featured resources and more. Sign up today to receive our FREE report on AI cyber crime & security - newly updated for 2024.
ITPro is a global business technology website providing the latest news, analysis, and business insight for IT decision-makers. Whether it's cyber security, cloud computing, IT infrastructure, or business strategy, we aim to equip leaders with the data they need to make informed IT investments.
For regular updates delivered to your inbox and social feeds, be sure to sign up to our daily newsletter and follow on us LinkedIn and Twitter.